Real-Time Fraud Detection with Graph ANALYTICS
Fight Fraud with TigerGraph
Powerful fraud detection software that catches fraud in real-time with advanced machine learning and deep link analytics
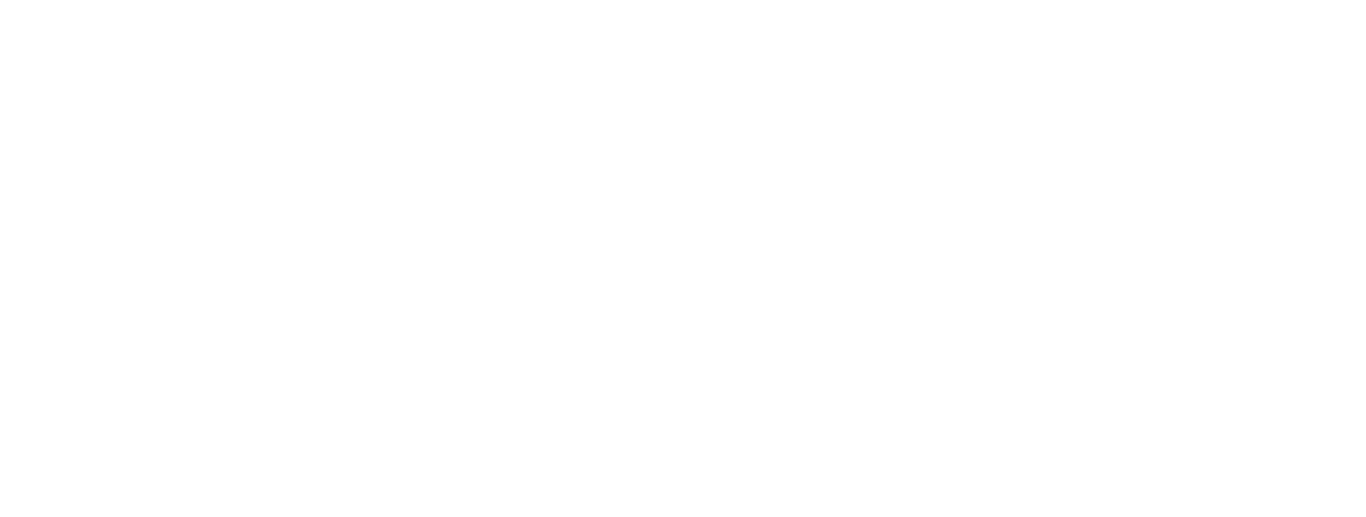
Fraud is Expensive and Becoming More Prevalent
Fraud is a growing problem in all industries, with eCommerce losses alone exceeding $57.8 billion in 2017. For every dollar involved in fraudulent transactions, the financial services industry spends $2.67 dealing with chargebacks, fees, interest, and labor.
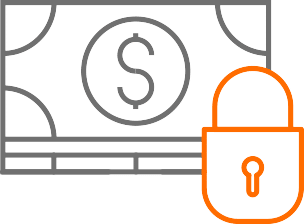
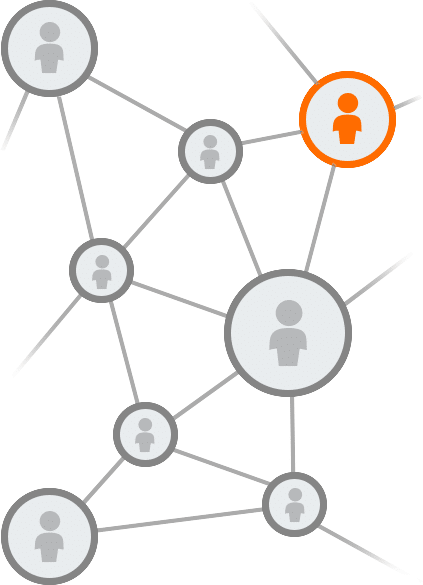
Fraudsters Are Becoming Increasingly Sophisticated
Fraudsters are getting more sophisticated over time, creating a network of synthetic identities combining legitimate information like social security or national identification number, name, phone number, and physical address. Legacy fraud detection systems are largely based on the analysis of the behavior of an individual business entity, such as a customer, citizen, device, doctor, or healthcare provider, and find unusual patterns in that behavior.
Fraud is perpetrated using fake accounts created with synthetic identities. Each individual fraud account looks and behaves much like a legitimate account, making detection much harder with traditional solutions. Detecting fraud requires going beyond individual account behavior, analyzing relationships among groups of accounts or entities over time, while often combining information from third party sources. Traditional fraud solutions built on relational databases were not designed to address this challenge.
How Does TigerGraph, a Native Parallel Graph Database, Help Find Fraud?
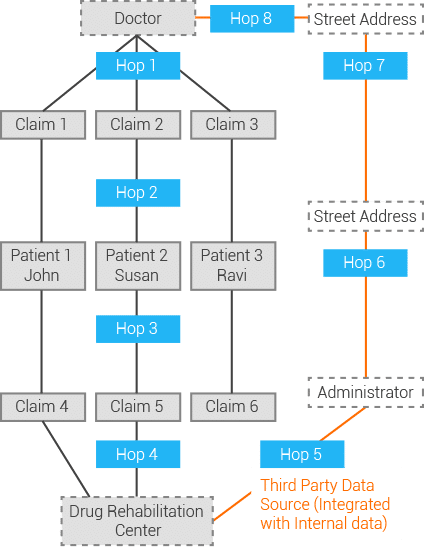
Fraud Detection with Deep Link Analytics
Take the example of fraud in healthcare, related to opioid addiction treatment centers. A provider is prescribing a large number of patients to one specific opioid or drug addiction treatment center. Traditional fraud solutions can’t find anything unusual based on the information available for the doctor as well as the treatment center.
TigerGraph’s deep link analytics taps into the enterprise knowledge graph from a third-party source – such as Thomson Reuters or Dunn & Bradstreet – to find all known administrators for the drug treatment center and their current and previous addresses listed in the public domain. One of the previous addresses for the administrator is very close to the address for the physician prescribing patients to the drug treatment center. In order to detect this hidden relationship between the doctor and the administrator, TigerGraph executes a query that goes eight hops across claims for patient visits and opioid treatment center claims by the same patients. Combining that with the third-party knowledge repository data such as addresses and phone numbers reveals the collusion. Traditional fraud solutions built on relational databases struggle to incorporate new data sources from third parties due to rigid schema and require computationally intensive database joins, rendering deep link analysis infeasible.
Accelerate Detection with Real-Time Analytics
Fraud detection is time-sensitive: every passing minute, hour, and day that fraud goes undetected results in increasing losses for your organization as well as for your customers or citizens. TigerGraph is purpose-built for real-time fraud detection to address this challenge. Let’s consider the example of China Mobile, the world’s largest mobile service provider serving over 900 million subscribers. China Mobile uses TigerGraph to detect phone-based fraud in real-time by analyzing the calling patterns of pre-paid subscribers. Subscribers are alerted in real-time of a potential fraudster call, with high-probability fraud calls being redirected to China Mobile’s call center for investigation.
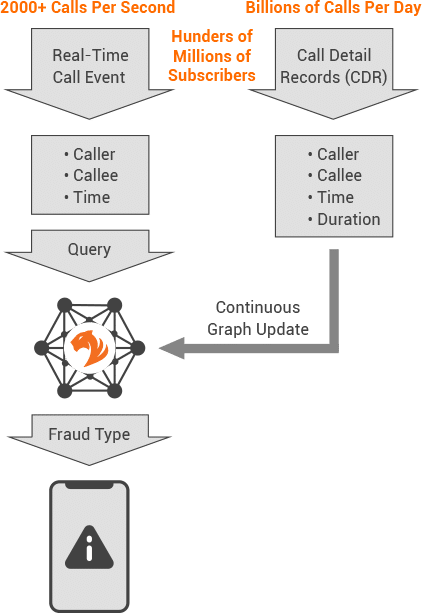
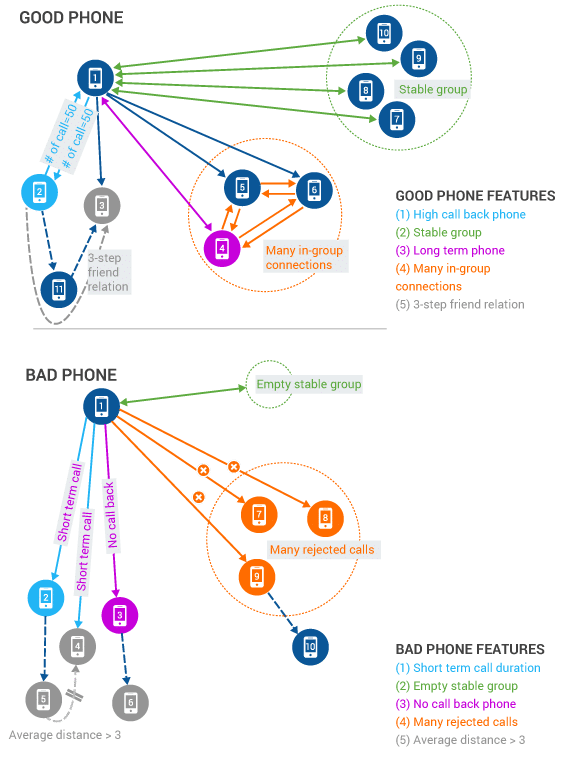
Improve Fraud Detection with Machine Learning
Less than 1% of the total call volume for telecom or claims data for healthcare and government benefits or payment transactions for financial services are fraudulent. This means that the machine learning models do not have sufficient training data with confirmed fraud activity to learn and improve their accuracy.
TigerGraph’s native parallel graph database is purpose-built to address this challenge. At China Mobile, TigerGraph creates over 118 features for each phone in real-time by analyzing relationships among subscribers over time, identifying a good phone owned by a regular customer compared to a bad phone suspected to be a fraudster’s.